Efficient and Consistent Joint Multi-Agent Prediction for Interactive Planning
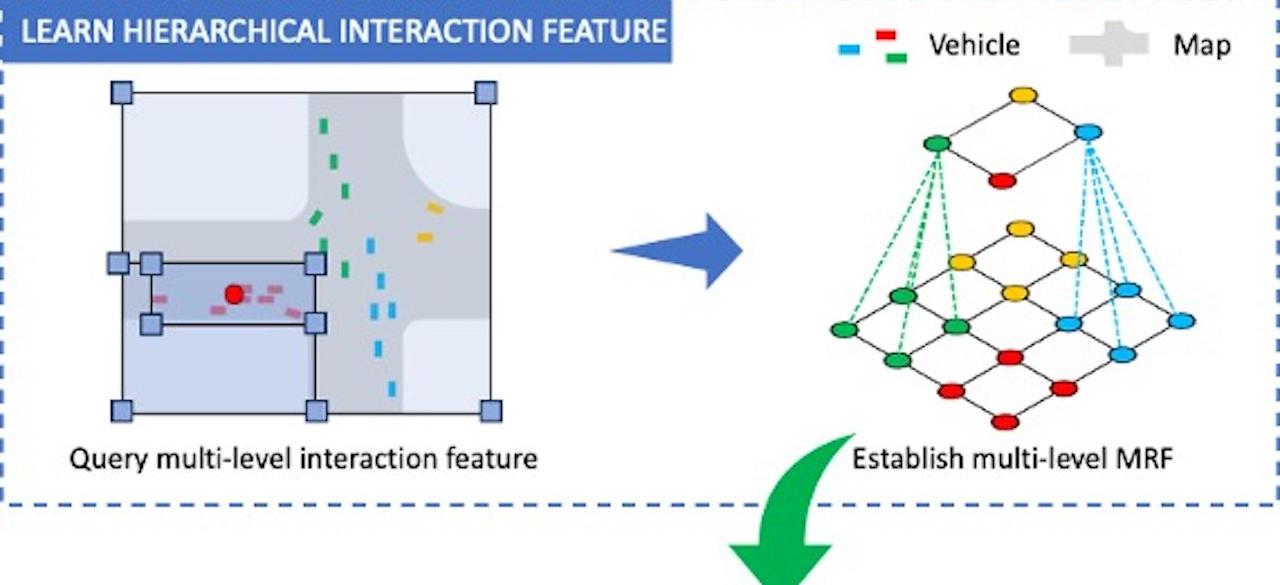
ABOUT THIS PROJECT
At a glance
In highly interactive traffic scenarios, it is crucial to accurately predict the joint future trajectories of multiple agents while considering the interactions among the agents over both past and future trajectories. In this joint multi-agent prediction task, consistency should be ensured in both the encoding and decoding stages. For scene encoding, the surrounding agents’ historical information should be encoded in a computationally consistent way to introduce proper inductive bias and improve prediction accuracy. For trajectory decoding, the future trajectories of interacting agents should be jointly predicted and consistent with each other (e.g., avoiding unrealistic overlaps). However, it is challenging to design a computationally efficient joint trajectory prediction model while ensuring consistency in both the scene context encoder and the joint trajectory decoder. In this project, we aim to tackle these open challenges and propose a joint multi-agent prediction framework that is computationally efficient and consistent. We also want to demonstrate that it can be used to enable efficient and safe interactive planning in challenging interactive scenarios, by integrating the joint prediction model with state-of-the-art interactive planning algorithms.
principal investigators | researchers | themes |
---|---|---|
Yixiao Wang | Multi-Agent Prediction, efficiency, consistency, Interactive Planning |